23 April، 2024
PH.D dissertation on “Deep Learning System for Detecting and Predicting Epileptic Seizures”
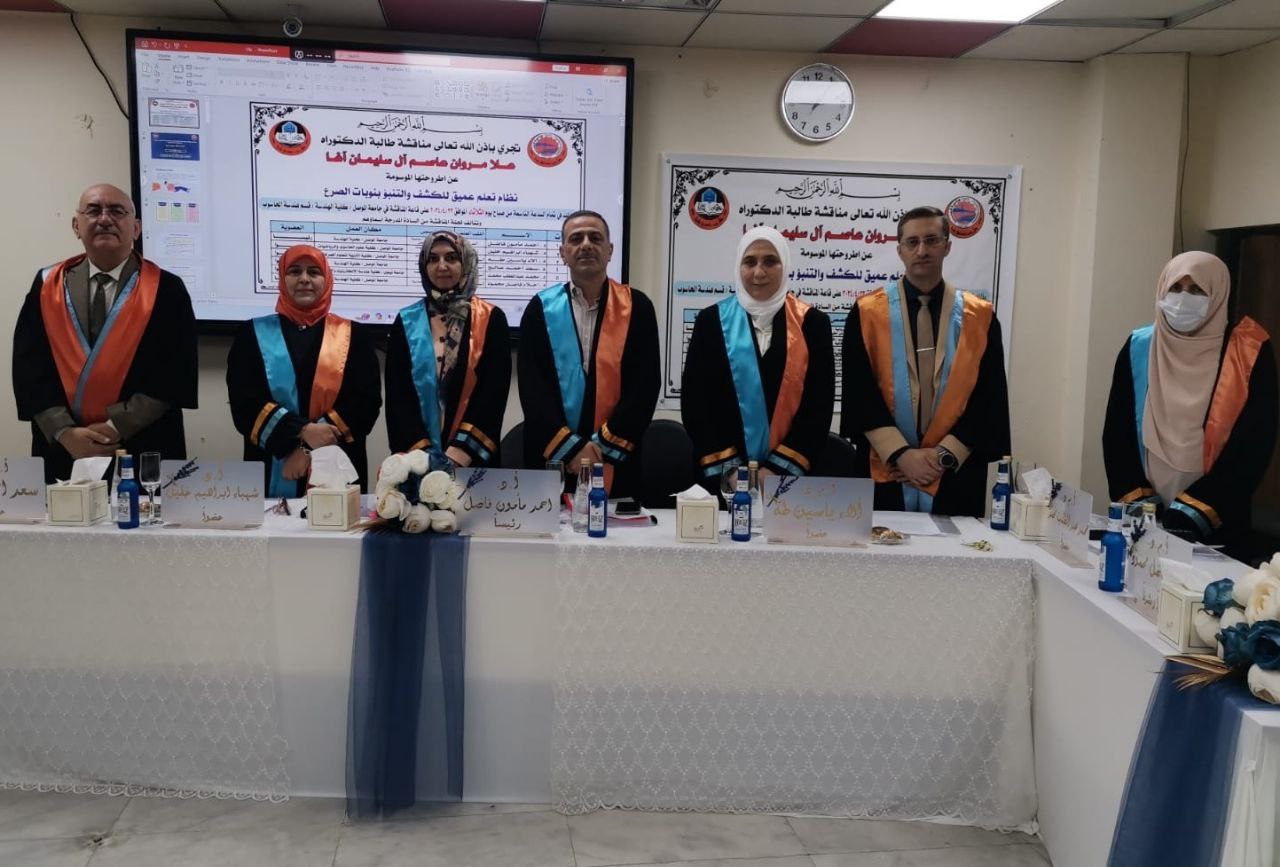
A PH.D dissertation was discussed in Department of Computer Engineering/College of Engineering at University of Mosul entitled “Deep Learning System for Detecting and Predicting Epileptic Seizures” submitted by the student (Ola Marwan Assem) on Tuesday, April 23, 2024.
The dissertation tackled from three perspectives: In the first perspective, related to diagnosis, the data was standardized regardless of the number of channels and sampling frequency, using the R programming language. The electroencephalogram (EEG) recording was also divided into frames (5, 10, 15, and 20) seconds, making the data compatible with deep learning requirements, as one million data samples were generated for epilepsy patients. In deep training, the spatial attention layer was added, which led to an increased diagnosis accuracy of 99.95, a sensitivity of 0.993, Specificity =1.0, Precision =0.993, and F1 score=0.993. And by comparing the accuracy for different frames created in this dissertation the highest accuracy was for frames of 5s. The proposed universal model can diagnose epilepsy with all types of EEG data regardless of the number of channels and sampling frequency used in various EEG devices. The second perspective is concerned with predicting the occurrence of a seizure before it occurs at an appropriate time and alerting the patient to take the necessary measures. A deep training system was created using ii LSTM, and a spatial attention layer was added to the model. The accuracy was 99.99, sensitivity was 1, and the false negative rate was 0 at a period of 20 seconds before the actual onset of the seizure. The final perspective is the design and implementation of a system for monitoring epilepsy patients according to the patient’s condition, which was implemented in Ibn Sina and Al Salam Teaching Hospitals by collecting patient data on symptoms and indicators of abnormal vital signs. Next, a code for each parameter was assigned in the patient profile to create a coding record for each patient to determine which device is suitable for optimal monitoring according to the patient code using Kmean clustering. The proposed real-time IoT system was tested with six patients at Ibn Sina Hospital and was able to predict a seizure 38 seconds before it occurred. The proposed system was well received by doctors in the epilepsy unit. The three parts of the dissertation were tested and implemented on an 11th Generation Intel(R) Core (TM)
i7-1165G7 processor clocked at 2.80 GHz.