29 April، 2024
PhD dissertation on “SIMD Architecture of Deep Convolutional Neural Networks (DCNNs) using Heterogeneous Hardware”
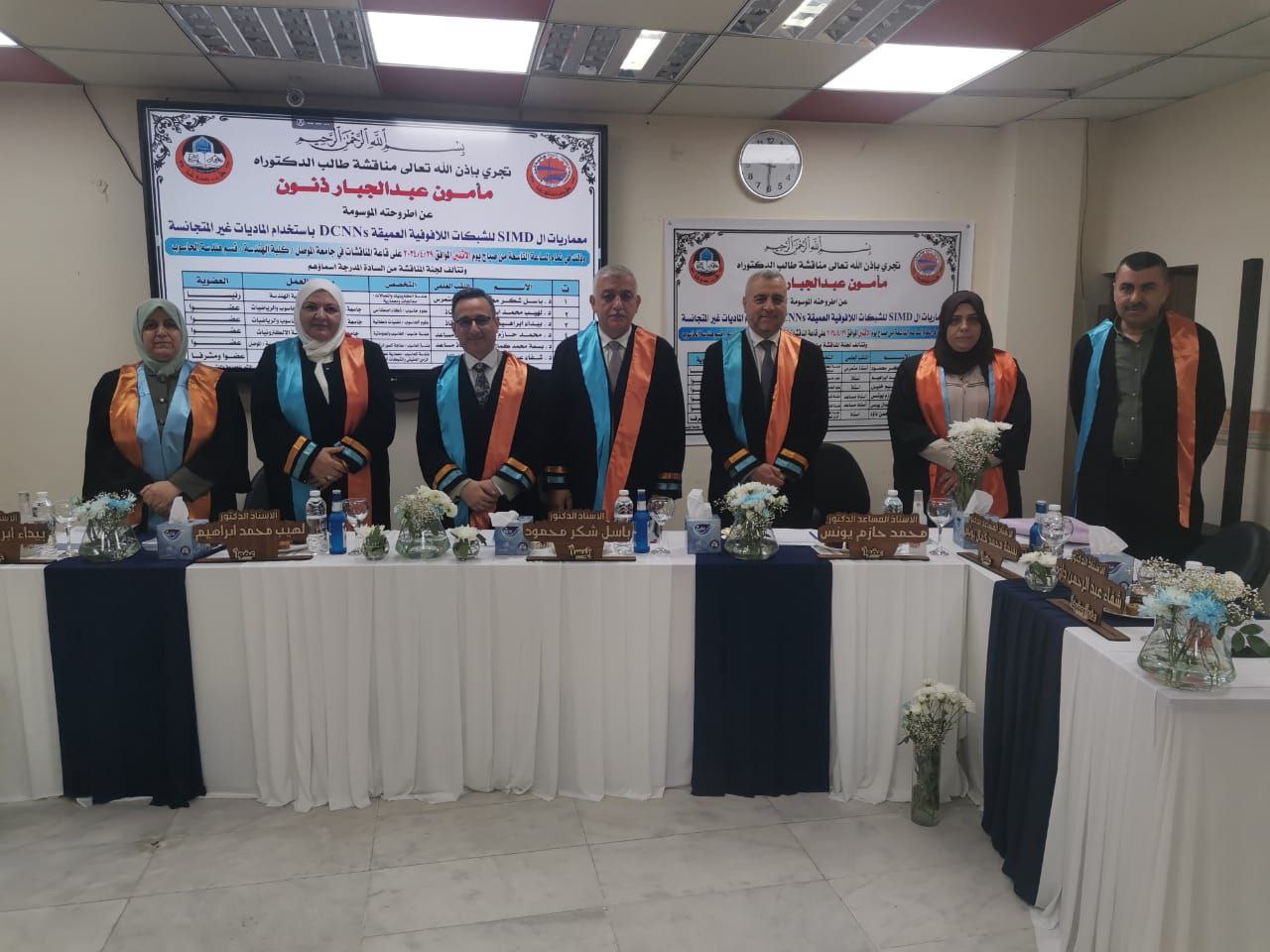
A PH.D dissertation was discussed in Department of Computer Engineering/College of Engineering at University of Mosul entitled “SIMD Architecture of Deep Convolutional Neural Networks (DCNNs) using Heterogeneous Hardware” submitted by the student (Mamoon abd al Jabaar Thanoon) and under the supervision Prof. Dr. Shifaa Abdulrahman Dawood on Monday, April 29, 2024.
The dissertation dealt with the investment of SIMD architectures in a new way to present a parallel deep system based on a proposed new deep network of limited size. This network has effectively contributed to represent and implement the SIMD parallel architecture in an environment with limited resources and low power consumption. The presented system is employed for detecting and classifying seven different ocular diseases in parallel within the same response time. SIMD’s parallel system architecture helps with the adoption of the multi-classification multi-training, based on the data parallelization and task parallelization approach, which facilitated and accelerated the system training process, as well as its effective contribution to improving the overall accuracy of the parallel system, which reached 91%.
In addition, the limited size of the SIMD deep system facilitates the process of translating it into a flexible and scalable embedded system that can be deployed in limited resources platforms such as Jetson platforms with a storage space not exceeding 4 Gigabytes and 128 Cuda-core only, while maintaining the overall accuracy of the system.
The emulation of SIMD architecture through a proposed new architecture based on a model parallelism approach also contributed to support the parallel implementation of deep learning networks on platforms with deficient power consumption, like IoT platforms, where the new architecture was implemented on the Raspberry Pi platform with power consumption not exceeding 3.65 watts while maintaining the accuracy of the deep network.