24 February، 2025
Master’s thesis by the student Raghda Azad Hassan
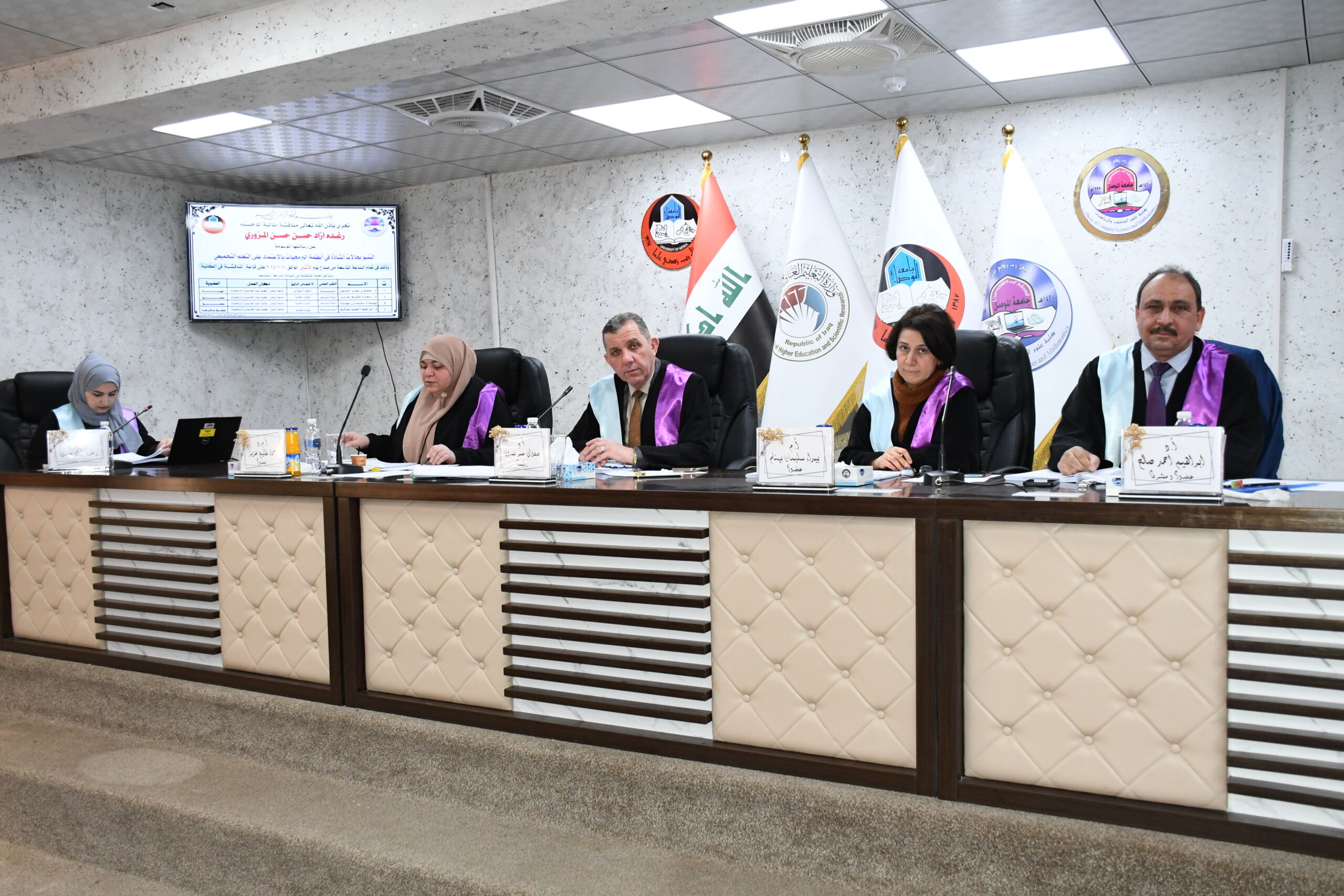
Discussion of the Master’s Thesis in the College of Computer Science and Mathematics – Software Department Titled (Prediction for Anomaly states in Software Systems Based on Ensemble Learning)
The Master’s thesis (Prediction for Anomaly states in Software Systems Based on Ensemble Learning) for the student Raghda Azad Hassan was discussed in the discussion hall at the College of Computer Science and Mathematics at the University of Mosul on Monday, February 24, 2025, under the supervision of Professor Dr. Ibrahim Ahmed Saleh
This study aims to improve the accuracy of predicting anomalies in software systems using ensemble learning techniques. Software is an essential element in various areas of life, as it contributes vitally to enhancing productivity and facilitating daily processing in the economic, health, educational and industrial sectors. With the increasing reliance on software, ensuring its quality and reliability has become an important goal. The proposed thesis presents an effective predictive model for detecting anomalies in software systems, which contributes significantly to improving the accuracy of prediction due to the complexity and diversity of data. Models based on ensemble learning are also characterized by their ability to integrate a set of different algorithms and processors, which leads to a significant performance enhancement. Through joint learning between different models, the efficiency of the prediction process can be improved. The proposed model relies on collecting and analyzing data related to the performance of various software systems, where three datasets were used: software anomaly data (SOFTLAB), performance anomaly data, and security anomaly data. The study relied on applying machine learning algorithms such as stacking, bagging, voting, adaptive boosting (AdaBoosting), and extreme gradient boosting (XGBoosting) to detect anomalies in the data. The results showed that the ensemble learning algorithms were effective in improving the prediction accuracy, where the AdaBoosting algorithm achieved the best performance on the 100% software anomaly dataset, while the Bagging algorithm recorded the highest accuracy of 99% on the performance anomaly. The proposed model also outperformed previous studies using the approved performance metrics.
The discussion committee was chaired by Prof. Dr. Safwan Omar Hassoun
and the membership of each of:
Assistant Professor Dr. Samaa Tali’ Aziz Member
Assistant Baidaa Suleiman Bahnam Member
Prof. Dr. Ibrahim Ahmed Saleh Member and Supervisor