5 May، 2024
master’s thesis of the student Zeina Nabil Jamil Al-Khatib
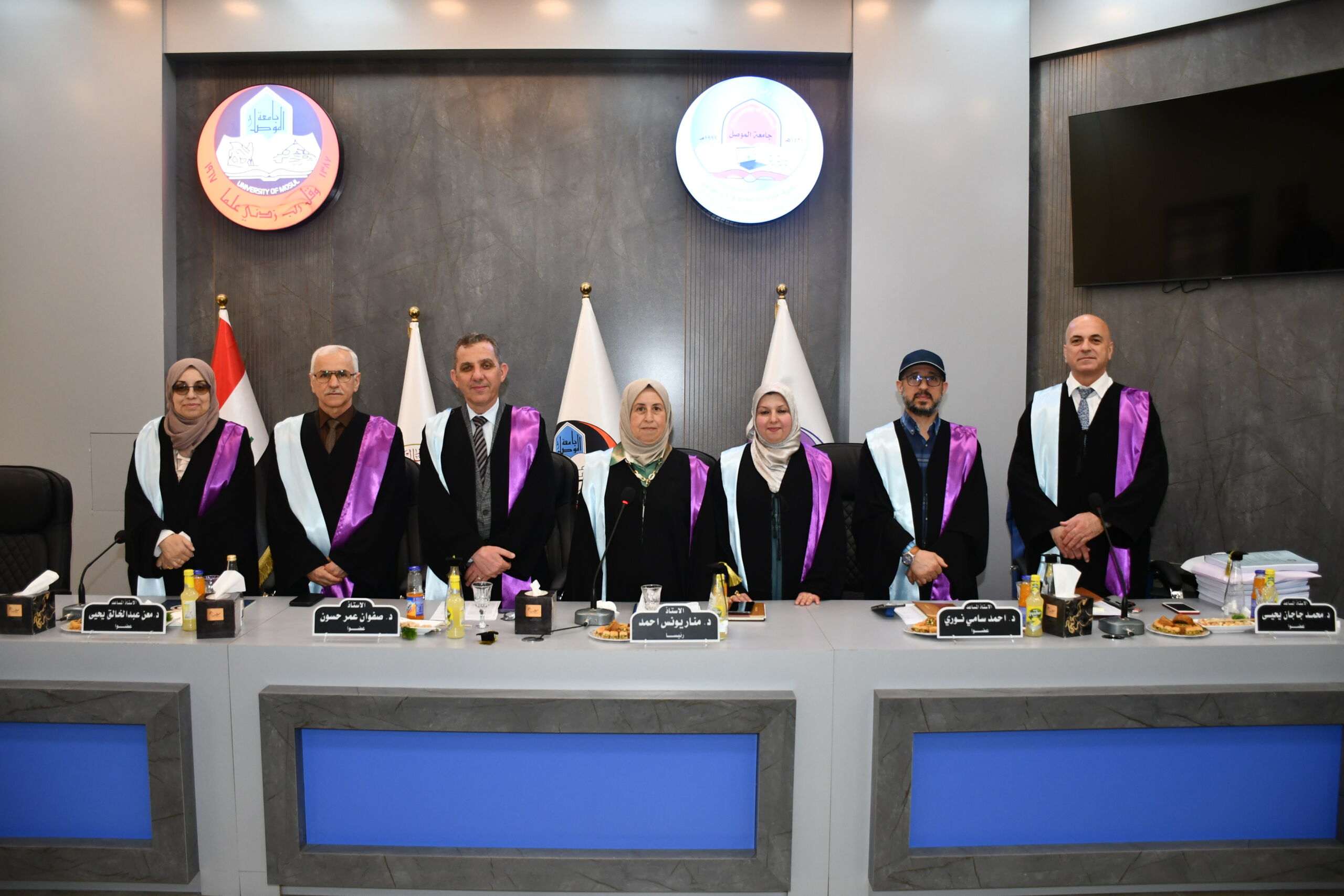
Defense of a master’s thesis in the College of Computer Science and Mathematics – Department of Computer Science
Titled Integration of Cloud of things (CoT) with Big Data for Telemedicine
Cloud of Things Integration with Telemedicine Big Data
In continuation of the scientific research movement and the follow-up and presence of the Dean of the College of Computer Science and Mathematics, Prof. Dr. Duha Bashir Abdullah
The doctoral thesis of the student Zeina Nabil Jamil Al-Khatib under the supervision of Prof. Dr. Duha Bashir Abdullah was discussed in the Cyberspace Hall of the College of Computer Science and Mathematics at the University of Mosul on 2 – 5 – 2024
The thesis submitted by the student dealt with
An innovative framework that combines the cloud of things, fog computing, machine learning and collective learning to handle big data generated by IoT devices to manage telemedicine. The proposed architecture harnesses emerging technologies, such as the Internet of Things and distributed computing, where tasks are divided between multiple parallel processing nodes and mobile applications, to improve quality of service (QoS) in real time. The framework combines fog computing and cluster-header-based load balancing, effectively improving latency and reducing network bandwidth and response time, and emphasizes the importance of ensuring system availability and continuity by leveraging cloud resilience, especially in cases of system inundation, along with hardware failure handling mechanisms.
The proposed framework provides the possibility of building an auxiliary cloud database. This includes storing pre-processed data in the cloud. The proposed framework has been applied and tested in predicting two different types of diseases: predicting gestational diabetes in pregnant women and predicting kidney disease through several scenarios. Experiments have shown good performance and efficiency in both applications. The improvement in prediction accuracy was about 6.5% when using pooled learning when using the proposed gestational diabetes prediction framework, and the improvement in energy use was about 87.01% when molten fog computing was used instead of cloud computing.
In terms of applying this framework to predict kidney disease, the proposed model achieved an impressive accuracy of approximately 99.953%, while the fog environment reduced latency by 31% and energy consumption by 75% compared to traditional cloud solutions.
These findings confirm the potential of the proposed framework as an excellent tool for healthcare in general and telemedicine and disease management in particular.
The discussion committee was chaired by Prof. Manar Younis Ahmed
Prof. Safwan Omar Hassoun Member
Assoc. Prof. Dr. Ahmed Sami Nouri Member
Assoc. Prof. Maan Abdel Khaleq Yahya Member
Assoc. Prof. Dr. Muhammad Jagan Younis Member
Assoc. Prof. Duha Bashir Abdullah Member and Supervisor